Recommendation 2: Harness Technology for Timely, Lower-Cost Evidence
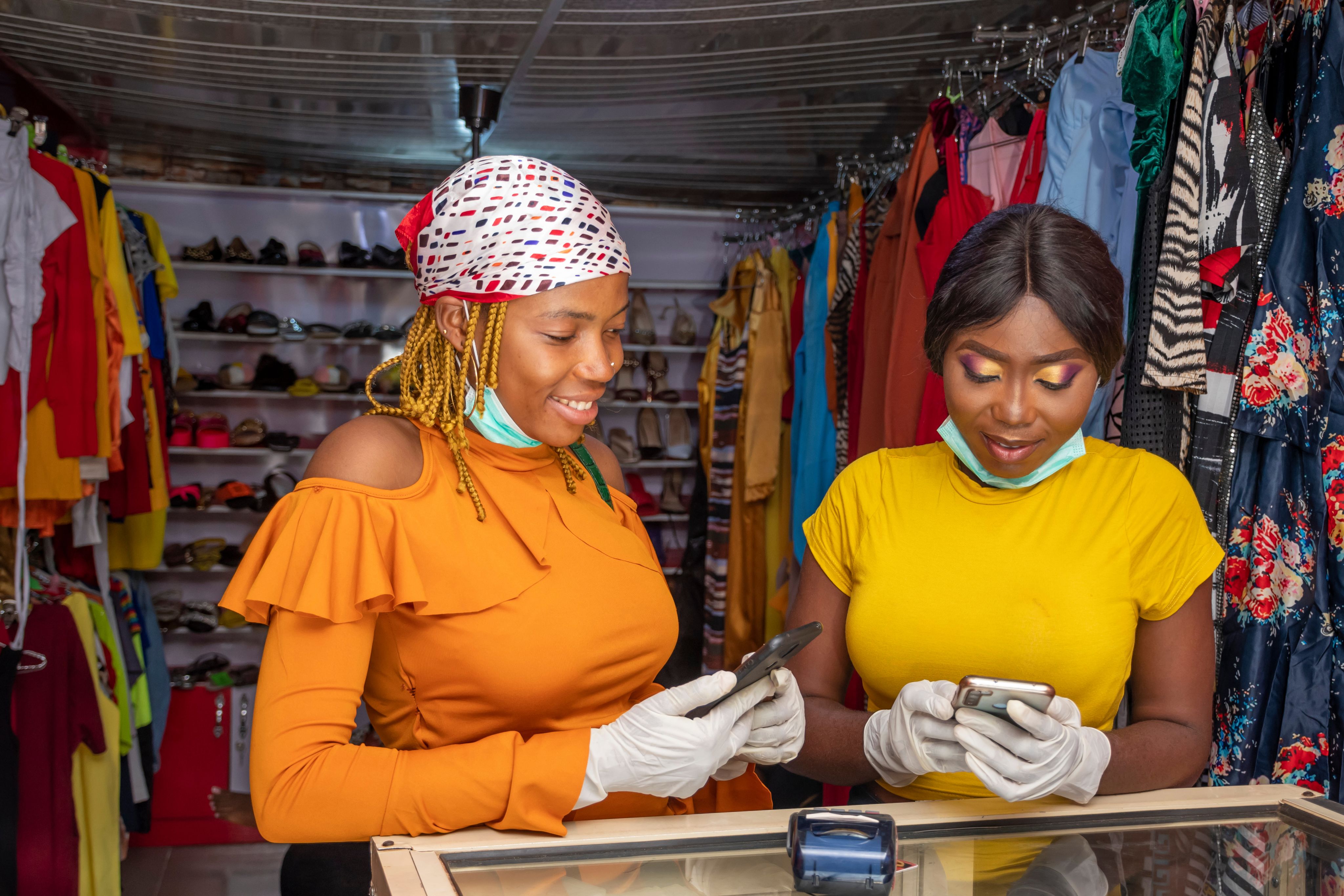
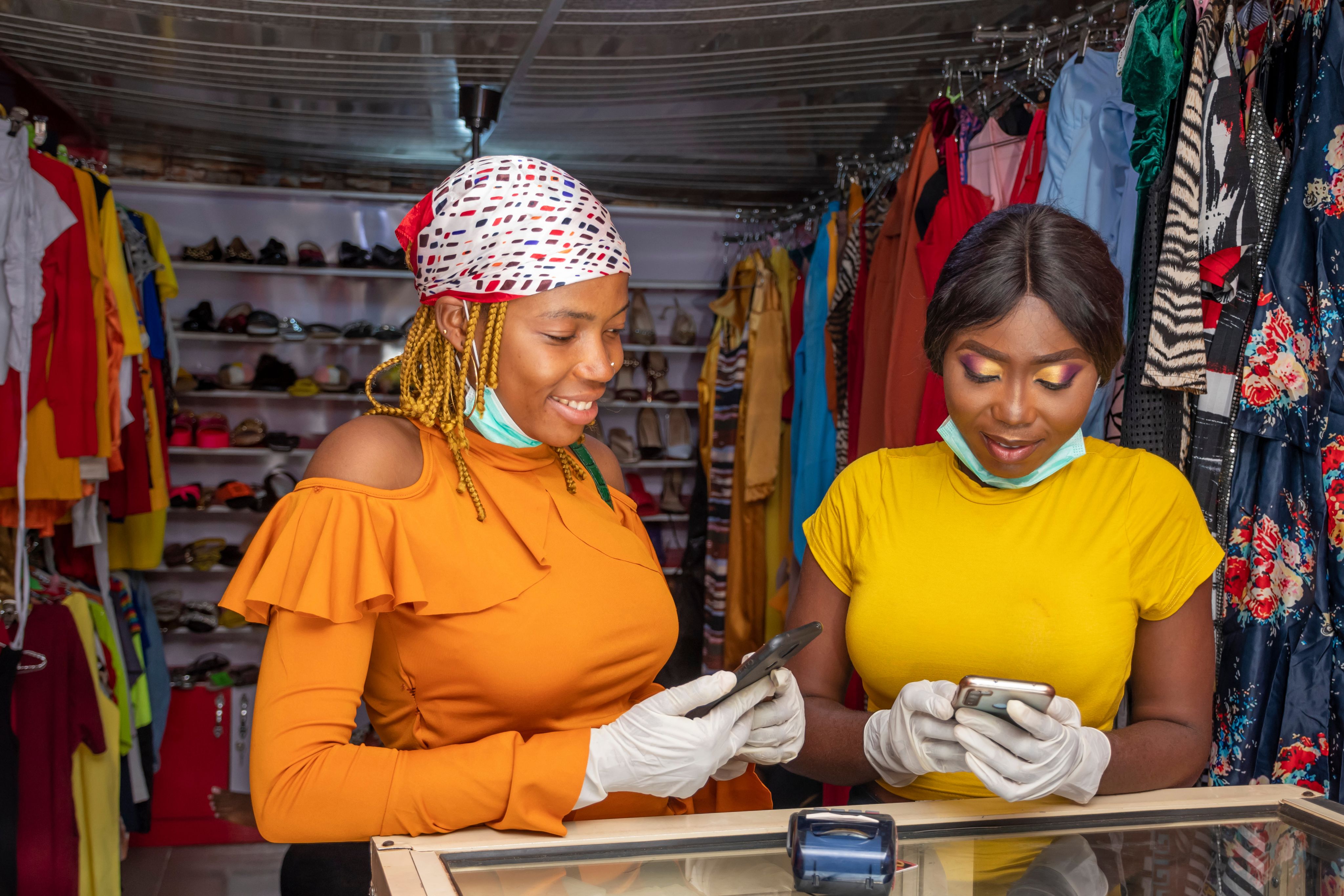
To generate faster, lower-cost, and more policy-relevant evidence, new developments and applications of analytical methods and data sources offer promising prospects.
Technological advances in Wi-Fi, cell phones, GPS, and satellite imagery have made gathering and sharing data much easier, and new types of software make this data easier to combine, analyze, and use. As discussed in section 1, five data sources in particular have ample promise for more relevant impact evaluations: geocoded survey data, administrative data, remotely sensed data, low-cost remote surveys, and big data (see Box 1 for more details).
But to harness the digital transformation and expand the use of these data sources, development stakeholders must commit to repurposing existing data and increase investments in capacity to do so. Administrative data can be used to unlock significant benefits for government functions, such as taxation and public procurement. Increased investments in the quality, regularity, and granularity of administrative data are high priority for routine decision making and use for impact evaluation (Glassman et al. 2014). Data quality can also be enhanced through partnerships with the broader “data for development” community, such as through developing a system of checks and balances with trust scores for administrative data.
To translate these data sources into policy action, researchers must prioritize the role of people, or human capital. More capacity is needed to validate large datasets through field surveys; provide technical assistance to manage, clean, and analyze big data; incorporate new data privacy and governance policies for legal use of private data; and continue to develop new methodological techniques. CGD’s Working Group on Governing Data for Development discusses how governments and multilateral organizations can strengthen data governance and protection policies for improved access and use, including a common approach to establishing the legality of cross-border data flows, more resources to strengthen domestic data governance regimes, and better data policy metrics (Development Data Partnership, a recent collaboration between international organizations and technology companies, provides a useful model for how to facilitate the use of private sector data for development research
Box 7. Examples of methodological innovations for rapid impact evaluation
1. Evaluations with multiple treatment arms: Many private companies have begun integrating continuous experimentation into their operations through A/B testing and other analyses (Chen 2020). As governments examine how to embed routine evidence into their own decision making for better outcomes, the widespread use of A/B testing to assess variations in program design is especially promising. For example, Banerjee, Alsan, et al. (2020) applied A/B testing to evaluate a COVID-19 prevention campaign in the Indian state of West Bengal, with several treatment arms receiving different messages. Since evaluating multiple treatment arms often requires usable administrative data and large sample sizes to detect impacts of incremental changes, greater support for data collection and infrastructure is critical.
Read more2. Adaptive/iterative evaluation: To enable real-time program adaptation, improve targeting, and inform rapid policy responses, evaluations can be set up to include multiple waves of data collection (through low-cost remote surveys, for example) and ongoing engagement with implementers. For example, an evaluation by Angrist et al. (2022) on the extent to which low-tech interventions limit pandemic-related learning loss in Botswana involved multiple rounds of data collection at four- to six-week intervals to facilitate program adaptation. Similarly, Caria et al. (2021) used “adaptive targeted experimentation” to assess the impact of labor market policies on Syrian refugees in Jordan by observing treatment outcomes over time and adaptively optimizing treatment assignment for participants. And Alvarez-Marinelli et al. (2021) fine-tuned an intervention to improve the reading skills of third-grade students in Colombia for each subsequent cohort and found that program effectiveness increased fourfold over time.
3. Technology to study heterogenous treatment effects: New ways to understand who benefits most from a given intervention can also be used to inform targeting, a key policy design issue (Vivalt 2015). For example, a study by Islam (2015) on the heterogeneous effects of microcredit programs suggests that effects on consumption vary across different groups of poor household borrowers, though Meager (2019) finds heterogeneity in effects across seven randomized microcredit experiments to be moderate.
4. Quasi-experimental methods: While econometric techniques to find “control” groups that are statistically similar to “treated” populations are not new, noteworthy techniques, such as synthetic controls and ML predictions, illuminate the increasingly sophisticated tools at researchers’ disposal (Athey and Imbens 2017; Abadie 2021). These techniques are often used in combination with novel data sources, including granular spatial data and large administrative datasets, to control for potential confounding variables at more specific geographic levels and assess relevant outcomes. “Surrogate” proxies are also a way to define outcomes of interest when using quasi-experimental identification strategies (Athey et al. 2019).
Source: Isaksson 2021.

